Data assimilation to the rescue: using forecasting methods to improve hydrological model structures for long-range projections
By Julien Lerat
In the forecasting world, data assimilation (DA) is everywhere. DA methods such as the Ensemble Kalman Filter can efficiently correct internal states of a model based on recent observations and generate optimal initial conditions at the start of a forecasting window. When these corrections are applied, DA can tell us when a model is not behaving as it should and provides remediation to this problem by updating the model states. Forecasting being a high-pressure job, most forecasters do not dwell on this point for long and move to the next forecast while discarding the previous DA corrections to replace them with more recent ones.
At another point in the modelling spectrum, hydrological models are used to generate long-term hydrological projections, for example under climate change scenarios. In this context there are no observations available, so there is no DA safety net to continuously correct states. This can lead to modelling errors propagating in time and, potentially, to underestimation of hydrological extremes. After several years spent on the development of a continental forecasting system (Vogel et al., 2021), we believe that this is unfortunate because considerable knowledge about model structure can be gained by analysing DA updates.
Reconciling the two concepts of DA and model structure improvement led us to the development of the Data Assimilation Informed Structure Improvement (DAISI) method. As shown in Figure 1, the method starts by assimilating observations (streamflow) in a hydrological model using the Ensemble Smoother (Step 1). The resulting updated states are subsequently analysed to develop correction to the state equations (Step 2). Finally, the corrected state equations are inserted into the model and the corrected model is run without DA over an independent period to check performance.
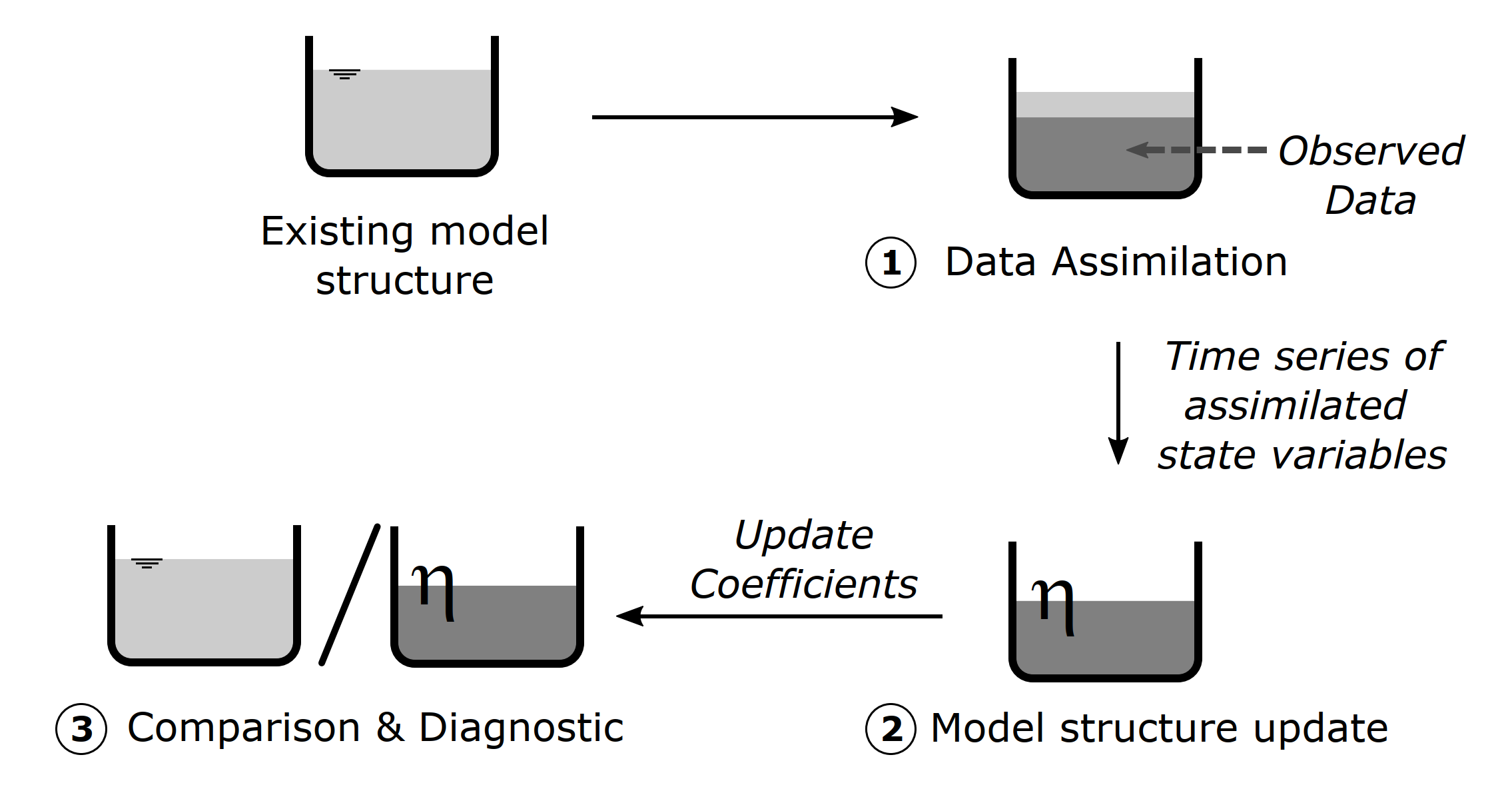
Figure 1 DAISI workflow diagram
In our paper (Lerat et al., 2024), this method was applied to the GR2M monthly rainfall-runoff model and 201 catchments in South-Eastern Australia. Figure 2 gives a quick overview of our results: DAISI state equation corrections lead to significant improvement for a range of performance metrics such as KGE. This improvement is particularly remarkable because performance is computed over an independent validation period, during which DA is not used to correct model states. Consequently, the new model obtained via DAISI constitutes a valid alternative to the original GR2M model.
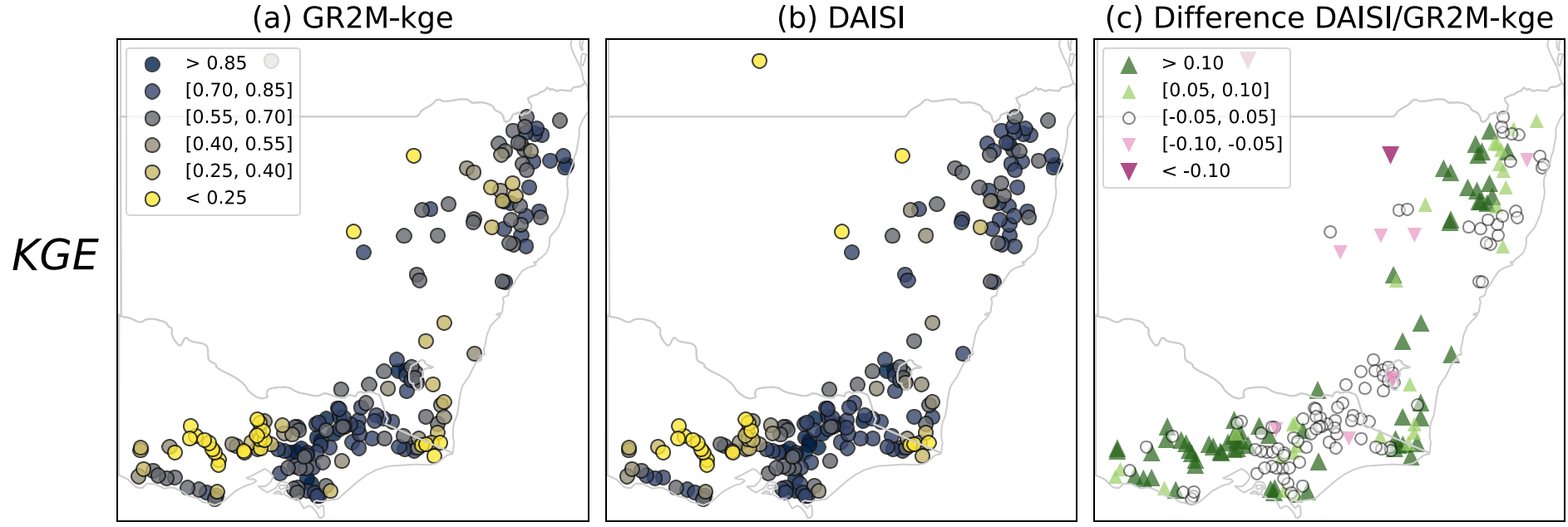
Figure 2: Comparison of validation KGE scores between original GR2M and DAISI corrected model structure
In summary, we believe that the DA methods typically applied to forecasting systems generate information that is currently underutilised in other applications like long range projections. We’ve shown that, if properly utilised, this knowledge can lead the generation of alternative model structures that bring significant performance improvement.
References
Lerat, J., Chiew, F., Robertson, D., Andréassian, V., & Zheng, H. (2024). Data Assimilation Informed Model Structure Improvement (DAISI) for Robust Prediction Under Climate Change: Application to 201 Catchments in Southeastern Australia. Water Resources Research, 60(6), e2023WR036595. https://doi.org/10.1029/2023WR036595
Vogel, E., Lerat, J., Pipunic, R., Frost, A. J., Donnelly, C., Griffiths, M., Hudson, D., & Loh, S. (2021). Seasonal ensemble forecasts for soil moisture, evapotranspiration and runoff across Australia. Journal of Hydrology, 601, 126620. https://doi.org/10.1016/j.jhydrol.2021.126620
0 comments