What is a good forecast?
Contributed by Tom Pagano, a HEPEX guest columnist for 2014
My interest in forecasts began in 1997 at the University of Arizona. I was studying how well weather models reproduced the effects of El Niño in the southwest US when a nearly unprecedented El Niño developed and captured everyone’s attention. It brought immediacy and focus to our work – people wanted to know about the possibility of floods; water was being released from dams, channels being cleared of debris, sandbags being laid. I was intrigued by the idea that nature would be giving scientists a closed-book exam; forecasts were hypotheses being tested.
A popular cartoon during the 1997-98 El Niño.
Around this time, Allan Murphy, a seminal researcher in the evaluation and use of weather forecasts, passed away. One of the Murphy’s most influential essays was “What is a good forecast?” He distinguished three types of ‘goodness’ (paraphrased in Beth Ebert’s verification FAQ)
Consistency – the degree to which the forecast corresponds with the forecaster’s best judgment about the situation, based upon his/her knowledge base
Quality – the degree to which the forecast corresponds to what actually happened
Value – the degree to which the forecast helps a decision maker to realize some incremental economic and/or other benefit
Consistency? Originally I was unsure when there would be a situation in which a forecaster’s beliefs differed from the official products. But years later, when I became an operational forecaster, I fielded questions from users along the lines of “Yes, the forecast is X, but what do you think is really going to happen?” Consistency is a great topic, worthy of its own discussion.
Murphy further unpacked Quality, listing attributes such as Accuracy, (lack of) Bias, Reliability, and Resolution as the desirable features of a forecast (described further in this HEPEX post on verification). Allan Bradley and co-authors later put these Quality attributes in a comprehensive framework for verification of ensemble streamflow forecasts.
The attributes of Quality are necessary but by no means sufficient for good forecasts. Murphy himself said (quoted in a recent HEPEX blog) “… forecasts possess no intrinsic value. They acquire value through their ability to influence the decisions made by users of the forecasts”.
However, anyone with a basic understanding of marketing would appreciate that the best designed or most effective products are not always embraced by consumers. Indeed Wang and Strong (1996) used marketing research techniques to study how consumers defined the quality of data and information (using ‘quality’ in a broader sense than Murphy, encompassing more of a sense of ‘fitness for use’). They had professionals and business students create and then prioritize a list of 179 Quality attributes:
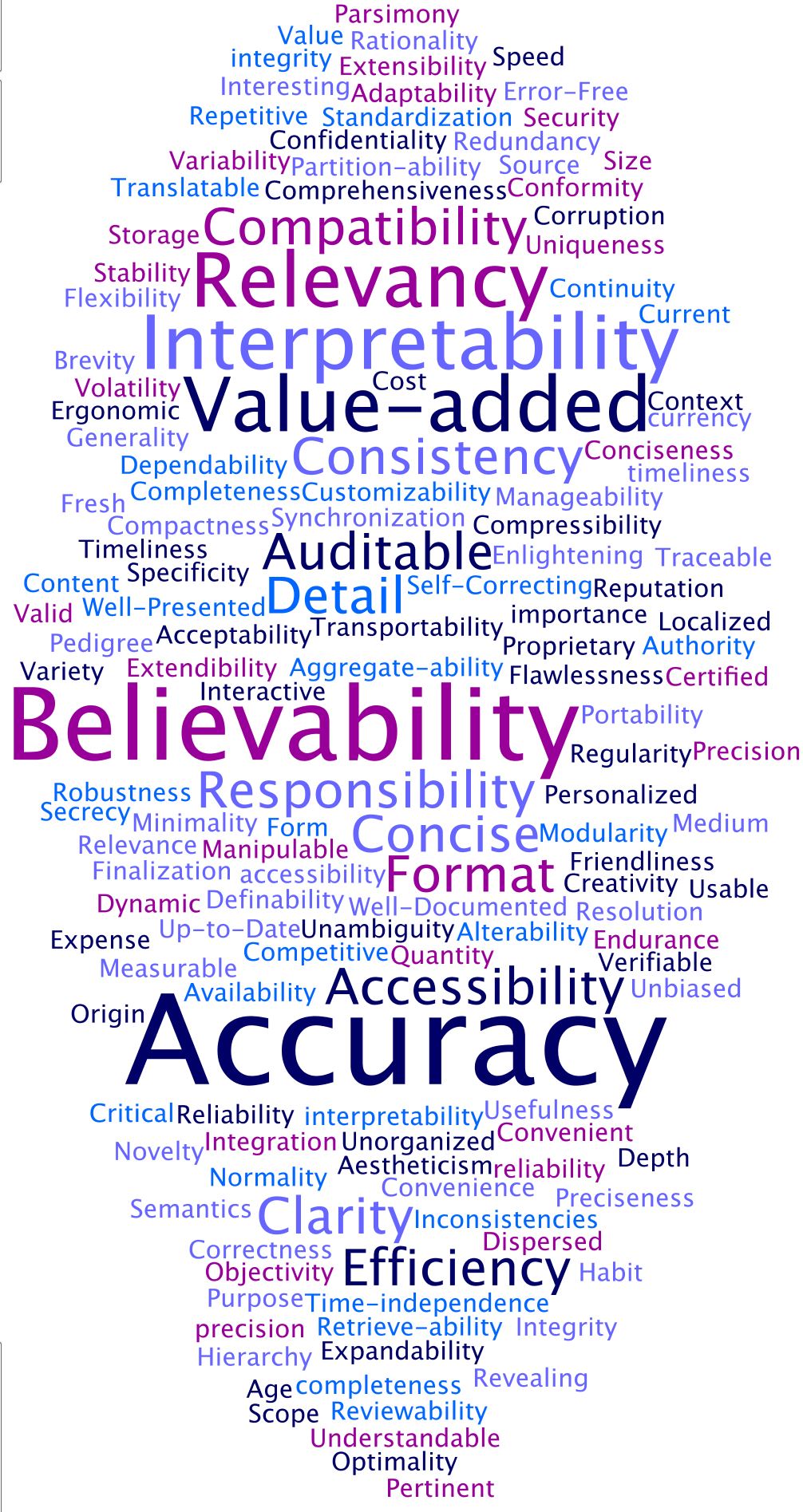
These were then grouped and prioritized into a subset of categories, such as Accuracy, Relevancy, Interpretability and Accessibility. A surprising result of their study was the importance of aspects such as Believability, Objectivity, and Reputation. If the goal is to have the customer use the information successfully, the customer must first believe that the information is trustworthy. The importance of credibility is illustrated in the emphasis that flood forecasting agencies place on preserving the reputation of their forecasts by, for example, forecasting correctly during fair-weather conditions and avoiding waffles (i.e. inconsistencies, as described in Florian Pappenberger’s article on the topic).
Others have created guidelines on measuring the goodness of forecasting services such as the World Meteorological Organization suggesting surveying user perceptions of Accuracy, Timeliness, Ease of Use, Accessibility, Added Value as well as Staff Responsiveness and Professionalism). Sometimes services are evaluated during external audits, such as the 1999 audit of the Australian Bureau of Meteorology or the Queensland Chief Scientist’s examination of flood warnings).
In these reports, along with surveys of the Information Quality research literature, five common themes on what makes a good forecast emerge.
Production (How the forecasts are created)
Produced in a cost-effective and efficient manner
Forecasts are reproducible
Created following professional Standard Operating Procedures whose documentation is available to the user
Production is operationally resilient (e.g. produced at the same time every day without fail)
Credibility (How the forecasts are perceived)
Honest, impartial and unprejudiced
Created and delivered by professional and responsive staff
Consistent with other sources or justifies why it is not
Accuracy (How good the forecasts are in a technical sense)
Low false alarm rate and high probability of detection
Relatively free from unconditional and conditional biases
Probabilistically reliable with an appropriate spread (narrow but not too narrow)
Verifiable (provide a time, location, and magnitude, not just one or two out of three)
Unambiguous and free of contradictions
Transmission (How the forecasts get to users)
Timely, in that it reflects the latest available information (is not stale) and arrives with enough leadtime for user to act
Available from a consistent source with a consistent and accessible format
Available with reliable and resilient access (e.g. accessible when power is out)
Forecasts maintain their message despite re-reporting through various sources such as radio, TV
Messaging (How the forecasts are framed for the user)
Clear and easy to understand
Complete yet brief and to the point
Communicates confidence/uncertainty clearly
Consistent message content (if different from last forecast, provide justification)
Conveys something that people can visualize (i.e. physical realism)
Meaningful units/Expressed in the user’s terms
Has personal meaning for those at risk
Relevant and specific to user vulnerabilities (e.g. locations, flood thresholds)
Provides options for action
Clearly scientists can contribute much more to the goodness of forecasts than just the technical aspects of accuracy. For example, Australian social scientists helped craft guides on how to word effective emergency warnings.
Do you feel that some under-appreciated attribute of forecast goodness requires more attention by HEPEX and the broader research community? Are there any aspects of goodness that are missing from the lists above? I welcome your feedback and discussion in the comments section below.
Next post: 18 April 2014.
Tom will be contributing to this blog over the year. Follow his columns here.
February 23, 2014 at 08:52
Tom
Seems to me that this discussion mixes up the meanings of forecasts and warnings. My understanding is that forecasts are based on science and that warnings advise people how to respond to a particular forecast. In my experience as a flood forecaster in Australia, engineers and scientists are continuously trying to improve accuracy and reliability of forecasts but the warning side of the equation has not kept pace. I think that relatively speaking, the science side of the equation is much easier than the social side as warnings have to be tailored to individuals as the impacts vary from person to person.
February 23, 2014 at 11:55
Terry, thanks for your post, I’m curious if you could suggest a “low hanging fruit” to improve the social side of warnings? Or is there some group who currently does that particularly well? Tom
February 23, 2014 at 12:31
As reflected in Alan Murphy’s distinction between accuracy/skill and usefulness is generally appreciated, but do we really understand or agree with what he meant by “consistency”? I am not sure myself. On one hand I agree with Murphy that if forecasters believe the probability to be 60% they should say so.
How the “proper” Brier Score seems to “know” that you are “dishonestly” not providing the probability you really believe in seems metaphysical – until you have worked yourself through the mathematics on page 287 in On the other hand, forecasters all over the world have the experience that they often must exaggerate the forecast probabilities to make people take the “right” actions.
Hedging towards the climatological mean is, however, a useful tactic in deterministic forecasting, even favoured by the “non-proper” RMS error score. If, in a late autumn evening, there is a 60% chance that a temperature of +3° C may drop to -5° C in case the low stratus clouds break up, I would seriously recommend a deterministic forecast of 0°, the weighted average.
It would, in my view, be optimal, not only statistically, minimizing the expected RMS error, but also from the average users’ point of view, minimizing their expected “pain”. But this deterministic forecast is, according to Murphy, “inconsistent” in the sense that I am fully aware that it will probably NEVER verify: it will most likely be either overcast with +3°C or clear with -5°C.
February 23, 2014 at 20:36
Anders, great comments. This reminds me of my experience with some fire managers trying to use some seasonal climate forecasts.
The forecasts of 1- and 3-month temperature and precipitation were produced using statistical models. However, the fire managers had experience using weather forecasts and tried to view the forecasts dynamically/synoptically. In other words, they were looking at the areas of high and low rainfall and were inferring the atmospheric circulation/pressure patterns. This is because they they were interested in extra variables that were not being forecast, namely wind speed, direction, humidity.
However, because the forecasts were created statistically, there’s no requirement that they have synoptic realism. Even though the average error is likely lower at the point-scale, the actual output of the statistical model is likely not going to resemble the observation at the pattern-scale.
I wonder if this falls under my goodness aspect “Messaging: Conveys something that people can visualize (i.e. physical realism)” Or would you give this another name?
February 23, 2014 at 15:42
This is an excellent discussion. I am an experienced forecaster, formerly, at the U.S. National Weather Service, Ohio River Forecasr Center. My experience with deterministic hydrologic forecasting has been that forecasters are reluctant to forecast the extremes and do hedge toward more climatic norms, as Anders suggests. Of course, in the long run, this pays off, but it does lead to providing less lead-time for extreme events than might otherwise be possible. Underlying this are: (1) will the forecast verify and (2) I don’t want to be an alarmist (and fail to be ‘right’). Too often, ad hoc, forecast adjustments are made that are ONLY defensible using the argument of ‘forecaster judgement’; unfortunately, many such adjustments have little scientific basis and can not be reproduced objectively.
February 23, 2014 at 23:12
For the last 30 years I have heard this: -Don’t hedge towards climate!
In my view, there is nothing wrong with deterministic forecasts hedging towards the climate! On the contrary, they should.
Indeed, hedging towards climate with increasing lead time is motivated not primarily because of the increasing lead time, but the increasing uncertainty due to the increasing lead time. If you are uncertain at D+1 you should hedge as much towards climate as if you experienced the same uncertainty at D+8.
But what about extreme events? Won’t the forecasts just be some useless “wishy-washy” scoring well with the RMS error verification? No, because extreme events with probabilities <50% (or perhaps <60%) do not qualify to be put in the deterministic, categorical “main clause” of the forecast, but belong naturally in the probabilistic “subordinate clause”.
“Moderate SW-ly wind with variable clouds, possibly local thunderstorms”.
Tom referenced in his column Wang and Strong (1996) who stressed the importance of ”believability” and ”reputation”. Nothing downgrades forecasters’ reputation and believability as “jumpy” forecasts. With non-extreme events mostly in the deterministic “main clause” and the extreme events in the probabilistic “subordinate clause” the psychological effects of forecast jumpiness will be highly reduced.
In the example above, the “main clause” can remain unchanged and provide a reassuring air of stability, even if the “subordinate clause” varies the degree of possibility and type of extreme weather.
Footnote: “Main clause” and “Subordinate clause” are in German called “Hauptsatz” and “Nebensatz”, in French “clause principle” and “subordonné”.