Should the forecaster modify an ensemble hydrological forecast? A case study.
Andrea is an operational forecaster at the US National Weather Service’s North Central River Forecast Centre. She writes about some very specific forecasting challenges that she and her colleagues are faced with on a daily basis.
Introduction
Devils Lake water levels have risen over 10 meters in the past 22 years. Due to natural variability in winter precipitation, the water level may further rise in spring as a result of melting of the snowpack in the lake’s basin. Such a rise causes significant problems for communities near its shoreline; measures may be taken to mitigate impacts if they receive a timely forecast.
Forecasting lake levels
Lake levels are forecast by the US National Weather Service’s North Central River Forecast Centre (NCRFC) in Chanhassen, MN. Water levels typically peak in July and the first spring outlooks are produced in January. The SNOW-17 models used were developed and calibrated by Dr Eric Anderson, formerly of NOAA’s Hydrologic Research Lab. Due to the confidence in the calibration, the Devils Lake model is usually “hands off” at an operational forecast office that typically makes routine modifications to model states. Prior to publication, forecasts are heavily coordinated between the U.S. Army Corps of Engineers, North Dakota State Water Commission, and the United States Geological Survey. This process is defined in a formal procedure, but the NWS has the final say.
The 2013 forecast
During the winter of 2012-13, data from independent snow surveys in the basin were inconsistent. The NCRFC modeled snow water equivalent was on the higher end compared with the surveys and ranked in the 90th percentile of the 60 year simulated period of record.
In late January 2013, when the frozen lake was at elevation 1451.5, the NWS issued a forecast showing a 10% chance of the lake getting back up to 1454, the 2011 record lake level. By mid-February, the model showed a 20% chance; by early March, 50%.
Starting with the January forecast, the coordinators disagreed on what the forecast should be:
“What are you using for initial soil moisture conditions? I’m having to push my model pretty hard to get the 50% elevation that high.”
“Has the precip since the last forecast been more than normal?”
“I don’t understand how we could get that much inflow with current conditions and average precip.”
“It seems like the NDSWC snow survey of 1 inch (perhaps more like 1.5 inches with the extra since Jan 28) is about half of what you are using. Which is right?”
“This past summer was not nearly as wet as 2008… Yet, you are forecasting similar inflow to 2009?”
So here is the real question: The coordinators think the forecast is too high; do we lower it, and how? The approach we took was to manually correct for biases in the model simulation record. We looked at streamflow exceedance graphics for several tributaries. One example, the Mauvais Coulee near Cando (mean daily flows) is shown in Figure 1. Green is observed and blue is the historical simulation; a perfectly calibrated model would have the green and blue exactly the same. For this location, the historical simulation shows a high bias for flows above 2200 cfs.
Modeled vs. Observed Mean Daily Flow comparison for Mauvais Coulee near Cando, North Dakota
Using this model bias approach, as well as pictures from the NDSWC of the snow cover (Figure 2), we determined we could remove some of the modeled snow water equivalent on the eastern side of the basin and hence lower the forecast. Afterward, exceedance of the 1454 mark was assigned a 20% chance; much lower than prior to the manual intervention.
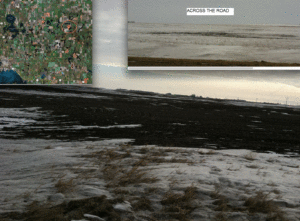
Figure 2: Snow Cover, Early March 2013, Devils Lake basin, picture courtesy of the NDSWC.
As a forecaster, I was uncomfortable making these changes. I had been monitoring the snow model since the beginning of the season and there were a few snowfall events in November that melted before winter really set in – and re-froze before making it through the modeled soil moisture. I felt confident in the current model states and previous model performance. However, the coordinators were in agreement that they thought our simulations were too high and they, too, are experts on Devils Lake.
What happened?
Following almost double the normal Spring precipitation, Devils Lake went up 2.5 feet to 1454.
Modeling a snowpack in the northern plains for spring melt runoff is extremely challenging. As happened in November of 2012, early snowfall events melted and the runoff didn’t make it into the system before it re-froze. This phenomenon can happen with a short term January thaw as well. The melt water is still there waiting to runoff in April, but no human observer can see it. I argue with people ALL THE TIME about the modeled snow water equivalent.
In retrospect, we would have been better off not tampering with the model. The model did a great job keeping track of the water available for runoff in the spring. We are forecasters, and hindcasting is only useful when it improves the chances of getting it right the next time.
June 15, 2015 at 07:50
Did it reach 1454 because of the “double the normal Spring precipitation”? Seems the thing to check would be the hands-off model simulation forced with observed rainfall. If that was just right, then I think you’re vindicated. But if it was too high, then maybe the experts were right. Otherwise I’m not sure if you can tell if someone’s getting the right answers for the right or wrong reasons.
June 20, 2015 at 20:20
Andrea and Tom,
I cannot see how any conclusions can be drawn from this single case, in particularly not with respect to the assigned probabilities. You would need about ten cases to draw any conclusions with respect to the deterministic forecast and perhaps 50 cases for the probabilistic forecasts. You would need almost 10 cases of 20% or 50% probabilities to make a fair estimayte if you or the system over- or underestimates the probabilities. And not least, the model should be unchanged during this time.
The above statements are based on conventional frequentist statistical procedures. It might be that a Bayesian approach might work better. It might in this case allow conclusions to be drawn in a mathematically consistent way with less data than the frequentist approach.
June 24, 2015 at 17:08
Thanks guys. The “forecasts” we issue for Devils Lake are probabilistic and not deterministic. I outlined one case of dozens where something similar happened — we are advised we have too much snow water in the model and pressured to lower it… With all the variables in hydrologic modeling, how do we ever truly know if we are getting the right answer for the right or wrong reasons? Since the forecasts include climate data from 1948-2012, then double the normal spring precipitation is included in the probabilities.
I wonder at what exceedance value does a probabilistic forecast “verify”? 25-75%?