Analogues are the new deterministic forecasts
by Marie-Amélie Boucher, a HEPEX 2015 Guest Columnist
According to Krzysztofowicz (2001), “Probabilistic forecasts are scientifically more honest [than deterministic forecasts], enable risk-based warnings of floods, enable rational decision making, and offer additional economic benefits.” More than 10 years later, I think most people (and especially members of the HEPEX community!) agree with this statement.
- But what about different types of probabilistic/ensemble forecasts?
- How do they compare to one another?
In the literature, it is very frequent to use deterministic forecasts as a benchmark for comparison with ensemble forecasts and show that ensemble forecasts outperform deterministic ones. It turns out that many end-users have been aware of the superiority of ensemble forecasts over deterministic forecasts for some time already. I don’t know how it is in your part of the world, but not a single operational agency I work with relies solely on deterministic forecasts.
At the same time, none of them use what I would (presumptuously?) call “real” hydrological ensemble forecasts based on meteorological ensemble forecasts. They rather use something in between: mostly different variants of the well-known analogues method.
On the choice of the most appropriate benchmark
Recently, Pappenberger et al. (2015) performed an exhaustive comparison between different types of benchmarks. They state that the choice of the most appropriate benchmark should depend on “the model structure used in the forecasting system, the season, catchment characteristics, river regime and flow conditions.” I would add that if you are using an operational forecasting system as a starting point for your research, one of your benchmarks should consist in this particular operational system. And, if I had the guts to do that, maybe I could cite myself as a bad example…
Hum…Well, okay, please just don’t tell anyone. In this paper, I show that ensemble forecasts outperform deterministic forecasts for the Gatineau River catchment managed by Hydro-Québec. But the thing is: Hydro-Québec has been using a sophisticated analogue forecasting method to produce their operational streamflow forecasts since the 70’s! Not deterministic forecasts! I was comparing my forecasts to the wrong baseline but I did not realize it at that time.
In my defence, I can only mention that none of my co-authors, including those working at Hydro-Québec suggested otherwise. Maybe everybody just had the same reflex? “Compare your ensemble forecasts to deterministic forecasts”.
Fortunately, one can learn from the past and I decided to always use the operational forecasting system (if there is any) as a benchmark for my ongoing and future projects, even if it means that sometimes, it might be disappointing (some analogue methods are really hard to beat!).
A small example for the Montmorency watershed
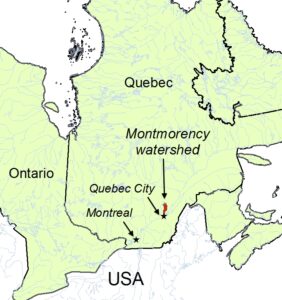
Figure 1: Localization of the Montmorency watershed
I want to show you a small, preliminary example, from an ongoing project on the Montmorency River (the tiny red watershed on Figure 1, which covers 1107 km2).
In this case, there is no disappointment: the good guys (ensemble forecasts!) still win (just be aware that these are not final results: the data assimilation scheme, for one thing, has not yet been implemented).
Here’s the example:
Currently, 1- to 5-day ahead streamflow forecasts are made available to the public and decision makers by the Centre d’Expertise Hydrique du Québec. Since 2013, these forecasts take the form of a mean forecast accompanied with a 50% confidence interval. The mean forecast (or 50% scenario) is in fact the deterministic forecast based on Environment Canada’s GEM atmospheric model. Precipitation and temperature forecasts are passed on to the distributed physics-based hydrological model HYDROTEL. The time step is 3h00 with a forecasting horizon of five days (120h00). The confidence interval is established based on the record of past forecasts and observations and it also depends on the season and on the forecasting horizon (see this post for more details). The analogues are obtained from a database that includes the errors between deterministic forecasts and observations from 10 watersheds, among which Montmorency. So it is not basin-specific as recommended by Pappenberger et al. (2015).
As an alternative, we propose using Environment Canada’s ensemble forecasts (also precipitation and temperature) instead of the deterministic forecasts. Each ensemble member is passed on to HYDROTEL, thereby explicitly considering the meteorological uncertainty based on current synoptic conditions and avoiding the use of analogues. The meteorological ensemble forecasts are also produced by the GEM model but with a different spatial resolution, different variants in the physics of the model and initial conditions.
Figure 2 shows hydrographs for both analogues and ensemble forecasts, for two different forecasting horizons (24h and 72h ahead). Analogues forecasts consist in the deterministic forecast dressed with a distribution with parameters that depend on past forecasts errors for the corresponding month. The time step is 3h00. The figure shows a short period of time during fall 2011, just to illustrate the case. The blue dots represent the ensemble forecasts and the red line is the observation.
Figure 2 : Example hydrographs for analogues and ensemble forecasts (blue dots=forecasts, red line=corresponding observation)
One can see that analogues are not that bad, especially for the 24h00 horizon. The mean CRPS (Figure 3, below) also confirms that, for the short horizon of 24h00, analogues and ensemble forecasts are quite similar. I also included the mean absolute error (MAE) on Figure 3, so you can see that analogues always outperform deterministic forecasts, as the ensemble forecasts do. Analogues therefore constitute a more challenging baseline than deterministic forecasts.
Figure 3: The mean CRPS and MAE as a function of the forecasting horizon
On the use of more severe benchmarks such as analogues
I see much effort being put on improving meteorological ensemble forecasts and still not that many end-users adopting them operationally. Many operational agencies instead construct ensembles from the deterministic meteorological forecasts and past climatology. But all those efforts put on improving meteorological ensemble forecasts should be beneficial to hydrology. Shouldn’t they?
In my opinion, using deterministic forecasts as the only baseline when assessing the performance of an ensemble forecasting system is akin to using the Nash-Sutcliffe criterion to assess the performance of a deterministic simulation or forecast. This criterion is based on a particular naïve forecast (the average streamflow) that is easily beaten compared to other types of naïve forecasts (ex. Schaefli et al 2007). For instance, the previous streamflow observation, used as a naïve forecast for a persistence-based criterion, is a much more challenging baseline.
Similarly, because they provide an estimate of the probability distribution, analogues are more difficult to beat than deterministic forecasts. In addition, there exists a wide variety of analogue methods, from very simple (like the ones in this blog) to very sophisticated (see, for example, Marty et al., 2012).
I know, for instance, that, in Canada, apart from Quebec and British Columbia, ensemble systems are only just emerging. In such a situation, a comparison between deterministic and ensemble forecasts is still relevant. But otherwise, I would like to see more studies comparing hydrological ensemble forecasts obtained from meteorological ensembles to severe benchmarks such as analogues. More such studies might convince end-users who are already using some form of uncertainty estimation to switch to incorporating meteorological ensemble forecasts in their forecasting process.
And you? What do you think? Is it really important for HEPEX to promote the use of meteorological ensemble forecasts in hydrology, or just any valid method of generating streamflow ensembles will do?
References
- Boucher M.-A., Anctil F., Perreault L., and Tremblay D. (2011): A comparison between ensemble and deterministic hydrological forecasts in an operational context, Advances in Geosciences, 29, 85-94, doi:10.5194/adgeo-29-85-2011.
- Krzysztofowicz R. (2001): The case for probabilistic forecasting in hydrology, Journal of Hydrology, 249(1-4), 2-9.
- Marty R., Zin I., Obled C., Bontron G. and Djerboua A. (2012): Toward Real-Time Daily PQPF by an Analog Sorting Approach: Application to Flash-Flood Catchments, Journal of Applied Meteorology and Climatology, 51, 505-520.
- Pappenberger F., Ramos M.H., Cloke H.L., Wetterhall F., Alfieri L., Bogner K., Mueller A. and Salamon P. (2015): How do I know if my forecasts are better? Using benchmarks in hydrological ensemble prediction, Journal of Hydrology, 52, 697-713.
- Schaefli B. and Gupta H. V. (2007): Do Nash values have value?, Hydrological Processes, 21(15), 2075-2080.
July 30, 2015 at 09:02
A meteorologists comment:
The well-known “buttefly effect” was discovered by Ed Lorenz around 1960 when he investigated the relative skill between statistically and dynamically based weather forecasts. Although they at the time were of equal quality (the computers were in their infancy) he reached the conclusion that the dynamical approach had more p o t e n t i a l to improve. And he was proven right. In line with these results statistically and dynamically based hydrological forecasts might be equally skilful today, but the dynamical approach s h o u l d have more potential to develop.
But the statistical methods are still very useful as a complement. During a coffeebreak in the ECMWF restaurant in the 1990’s Lorenz told me a story he had heard from a Norwegian colleague, Arnt Eliassen.
The birds had a competition about who could fly the highest. They set off, but one after the other had to give up. Finally, the Great Eagle was the only one left, but at some stage also he had to stop. In that moment a little sparrow who had hid itself in the ealgle’s feather sprang out and managed to reach an inch or two above the eagle, and thus come out as the winner.
-The eagle is the dynamical numerical prediction system, said Lorenz and the sparrow the statistical post-processing.
July 30, 2015 at 22:17
Thank you for your comment and for the bird analogy. In another project I am working on, the sparrow (sophisticated analogue/statistical method) is indeed the winner in the competition with the Great Eagle (meteorological ensemble forecasts). For a number of reasons it was not possible to use this particular example on the blog but hopefully it will eventually be published in a journal.
I also received a relevant comment by email and I would like to report it here. The person disagrees with me and says that it is still very important to use deterministic forecasts as a benchmark because although many operational agencies are producing ensemble forecasts, they are not always considered in the decision-making process. He says that (1) most decision makers still have a deterministic perspective, wanting to know THE best scenario and (2) most stochastic optimization models used in the hydropower sector operate in a deterministic framework. So the details on how precisely you obtain the ensemble of streamflow forecasts is of no consequence if in the end the optimization model is deterministic.
I think it stresses (once again!) the importance of multidisciplinary collaboration, in this case between forecasters, decision-makers and operations research analysts.
July 31, 2015 at 14:48
Sweden in the 17th century, had a wise stateman, Axel Oxenstierna, who is famous for having told his son: “Do you not know, with how little wisdom the world is governed?”. The fact that “most decision makers still have a deterministic perspective, wanting to know THE best scenario” among many to chose from, is not only the case in hydrometeorology but unfortunately in all straits of life, in politics, military, economy… That’s why the world looks as it does.
The positive outlook is that there are a lot of book published, and more to come, about rational descion making, so if we are lucky things will improve before the end of this century – or the next.
I once escaped to be killed in a car accident just because I applied a probabilistic thinking. The pessimsitic outlook is that it will take around 100 000 years before all those who are bad at probabilties have no longer a chance to pass on their genes.
November 23, 2015 at 06:06
Marie-Amelie, thanks for a great post — quite provocative. The issue of baselines or benchmarks is important, and I can’t count the number of studies I’ve seen that use a simple climatology as a reference forecast when far more demanding and appropriate benchmarks exist. I do think the deterministic benchmarks are interesting and appropriate, even when ensemble ones exist, simply because many agencies (both forecasting and user) in the US still depend on that standard. Some RFCs in the US have advanced preliminary forms of ensemble forecasts, but the official deterministic ones are still most used by water agencies and other users. It’s a slow transition.
As a co-chair of HEPEX, I wouldn’t say there’s any less interest in ensembles constructed through analoging than through a sequence beginning with met. forecasts. The whole point is to quantify forecast uncertainty, and analogues can be a valuable and often efficient strategy. In addition, users often respond well to analogues because they incorporate something from the past with which the user is familiar, and that can aid the forecast communication and interpretation aspect.
November 26, 2015 at 21:07
Thank you for your comment Andy. I think that not all end-users are at the same point right now regarding hydrological forecasting… You and my colleague (the email I mentioned in a previous comment) are probably right that deterministic forecasts still remain a useful benchmark.
Analogues are definitely a valuable form of ensemble forecasts and I totally agree on their efficiency. That is precisely why I consider that they should be included more often in comparative studies. It could be “meteorological ensemble- based forecasts” vs analogues vs deterministic forecasts.
I also agree on the intuitive appeal of analogues for many users… But I remain eager to see more operational uses of meteorological ensemble forecasts in hydrology. There are some examples (EFAS, for one), but still not that many.