Assimilation of in-situ and satellite data in hydrological predictions – Can we add value?
Contributed by Jude Musuuza (SMHI).
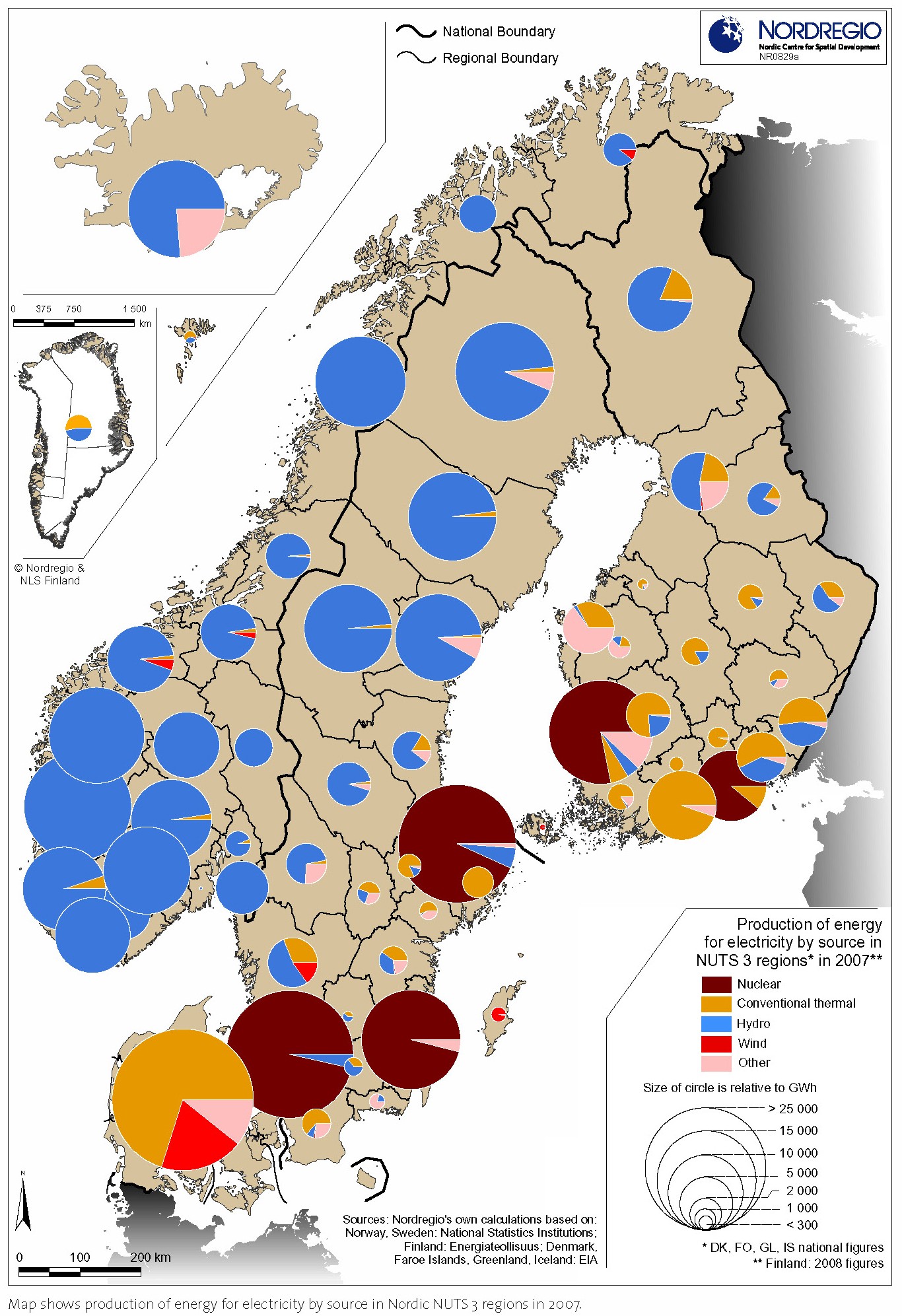
Production of energy for electricity by source in NUTS 3 regions in 2007
The increased focus on satellite missions in recent years has resulted in a rich source of valuable Earth Observations (EO), in terms of spatial coverage and temporal frequencies that are impossible to achieve with direct measurements. Such observations have been used in various disciplines, including also hydrological modelling, for instance to improve process understanding through tailored model parameterisation and performance assessment. In addition EOs have been used to (better) initialise the model states which further affect hydrological forecast accuracy.
The Nordic rivers have some of the highest installed hydropower capacities in the world, and hence process understanding is critical with a consequent economic impact. Most catchments are snow-dominated and the stream flow regimes are spring-melt dependent; most of the water is held in the snow-pack during the late autumn and winter months, and melts later in the spring/summer months.
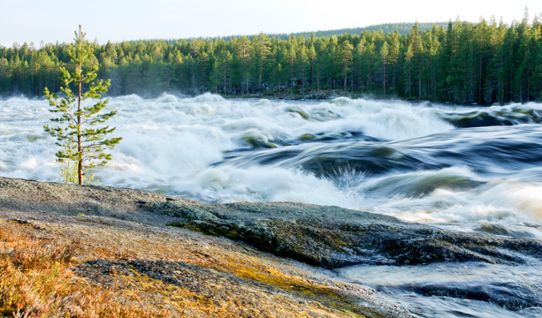
Spring flood in Vindel river in the north of Sweden. The river extends from the district around the Norwegian border to run into the Umeälven river just before it reaches the sea (source: SMHI archive).
SMHI has been in strong collaboration with water authorities and hydropower companies. One of the targeted investigations has been on improving hydrological predictions through assimilation of both EO and local in-situ data.
We therefore investigated the improvement in hydrological model performance by assimilating such products individually and in combination (see our recently published article Musuuza et al., 2020). This computationally expensive experiment was based on different combinations of two in-situ datasets (discharge [Qob] and reservoir inflow [INFLOW]) and four EO products (MODIS potential [PET] and actual [AET] evapotranspiration, CRYOLAND fractional snow cover [FSC] and snow water equivalent [SWE]). The 6 datasets were arranged into 57 unique combinations, giving a total of 63 (57+6) assimilation experiments.
We searched for answers about the combinations of the EO and in-situ datasets that add value to our model predictions. The data were assimilated using the ensemble Kalman filter, while model performance gain was based on the Kling-Gupta Efficiency and its three decomposed terms (ρ for timing, β for volumetric error, and α for variability) using as reference the observed records and benchmark open-loop simulation.
Assimilation of individual products
We found (Figure 1) that the assimilation significantly improved the modelled SWE and PET. However it should be mentioned that the passive microwave snow water equivalent products in mountainous terrain and coastal areas or when snow is deep show are generally inaccurate. The MODIS evapotranspiration products are also known to be biased; however it is possible here that the model was unable to properly represent fluxes in those regions and hence the gain in performance.
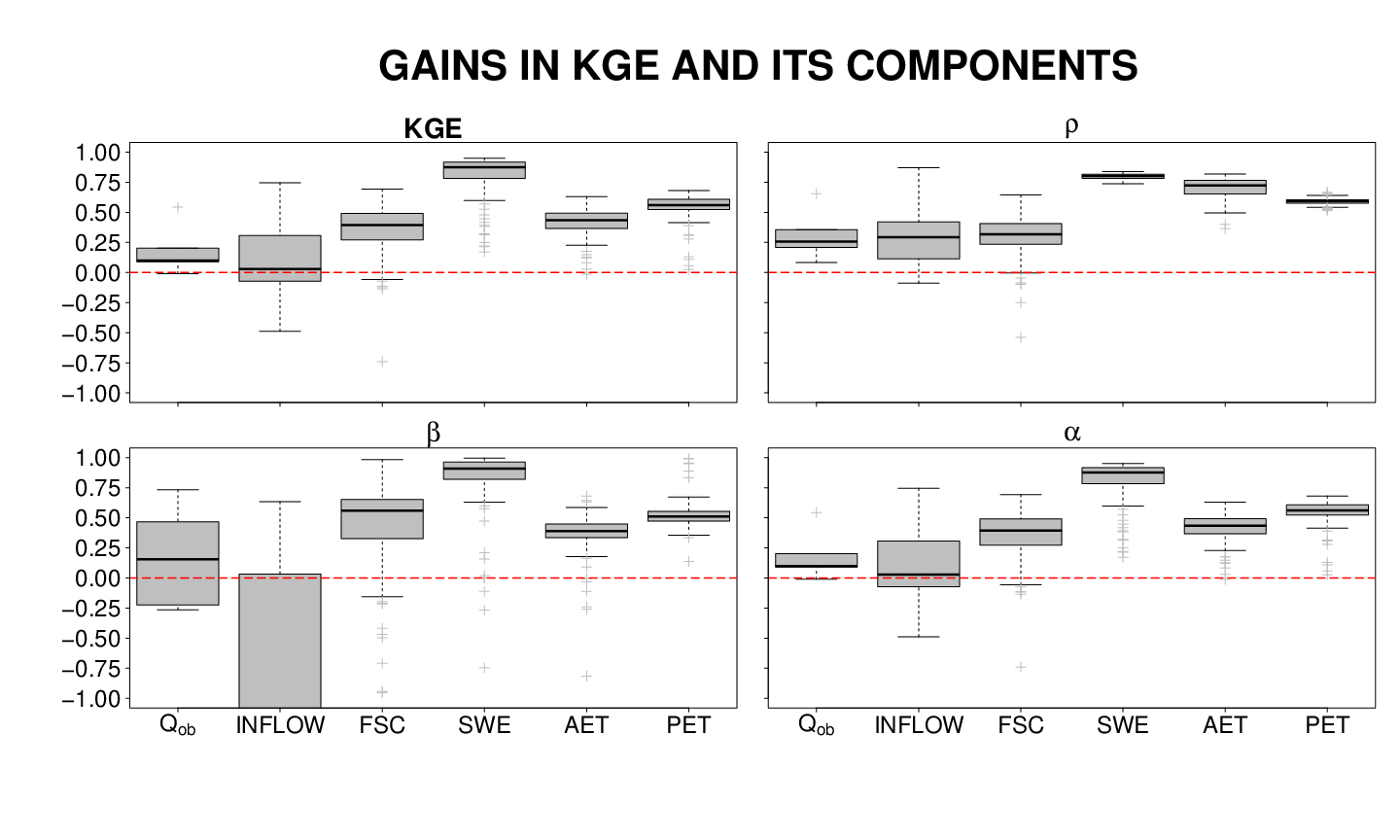
Figure 1: Gain in model performance after assimilation. Red lines represent the open-loop simulation with reference zero gain (source: modified from Fig. 4 in Musuuza et al., 2020).
Assimilations of product combinations
We then focused on the variables that water authorities and hydropower companies use in decision making: Qob and INFLOW. The 63 assimilations used these as the target variables. We defined informative combinations (green circles in Figure 2) in which the assimilations achieved performance gains in both target variables. The informative combinations are listed in Table 1.
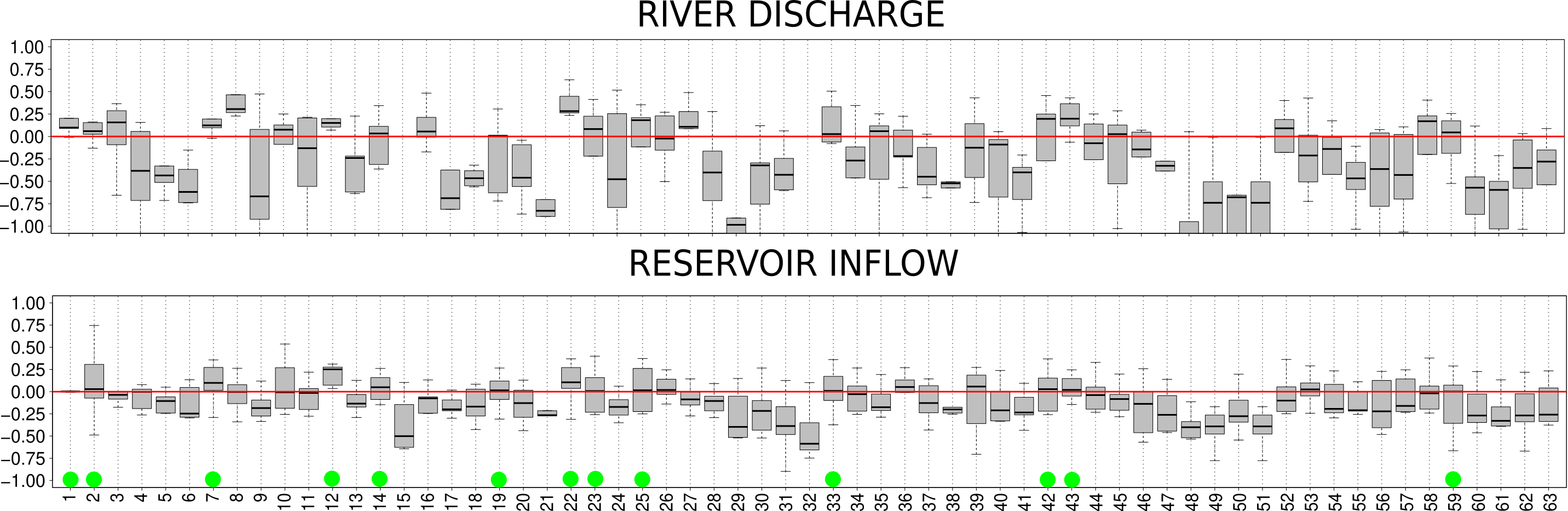
Figure 2: Gain in KGE from the different assimilations. Red lines are the open-loop simulations (source: modified from Figures 5 & 6 in Musuuza et al., 2020).
The products can be ranked based on the number of times they appear in informative combinations. Our investigation showed that the performance ranking is: INFLOW, Qob, FSC, AET, SWE, and PET. The in-situ observations outperformed EO datasets, partly because the observations aggregate all the information from their respective drainage areas, including the system memory, whereas the EO datasets are instantaneous “screenshots” that also depend on the retrieval algorithms. All the informative assimilations, except Qob alone and combinations containing PET, resulted in gains during the snow-melt months. Essentially, those assimilations have the potential to provide initialisations for reservoir inflow forecasts for use by the hydropower companies.
Table 1: The informative combinations (Table 4 in Musuuza et al., 2020)
In summary, the Ensemble Kalman Filter needs 100 members to deliver stable solutions with HYPE, the model used in this study. The assimilation of the MODIS evapotranspiration data-sets, especially the PET, did not improve the estimation of the stream discharge and reservoir inflows, our two target variables. The assimilation of in-situ measurements outperforms satellite data, possibly due to the aggregated catchment information included in the in-situ observations. We also obtained 13 combinations of the different data-sets, whose assimilations improved the model predictions of the target variables. In most of these, there were improvements in the prediction of reservoir inflows during the snow-melt months. There is big potential in using such data assimilation to provide initialisations for snow-melt forecast models, used by hydropower companies in the north of Sweden.
Find out more:
Musuuza J., D. Gustafsson, R. Pimentel, L. Crochemore, I.G. Pechlivanidis (2020), Impact of Satellite and In Situ Data Assimilation on Hydrological Predictions. Remote Sens., 12, 811, doi: 10.3390/rs12050811
0 comments