Where do babies come from?
Contributed by Maria-Helena Ramos and Florian Pappenberger
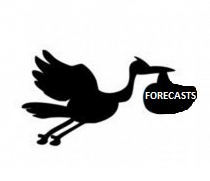
Please note: Stork population and Weather Forecasting Skill is significantly positively correlated!
In hydrology, many operational forecasters are usually receiving weather forecasters from a meteorological service without in-depth explanations regarding where these forecasts come from (there are a few laudable exceptions usually in organisations where the hydrological and weather forecast are issued by the same institution or where a concept of Public Weather Advisors exists – see for instance in UK).
We can imagine that weather forecasters are so used to their forecasting practices that they forget that users may not be acquainted with numerical weather prediction models and on how models create forecasts. In addition they have to deal with a large user community from forecasts on TV or radio, to energy companies, public health (e.g. heat waves) to flood forecasting centres.
A short course (or some hours of internet navigation) can fill the knowledge gap and any user of weather forecasters can quickly end up with the basic concepts behind computational fluid dynamics, 4D variational data assimilation or isobars charts – out of which she or he have to distil the knowledge which is actually required.
The question then seems to be solved. But then the operational hydrologist discusses his/her recent acquired knowledge with the meteorologist who says: “Well, you know, model outputs are not all. Our forecasts are then expertized and forecaster’s ‘best judgement’ is used to produce the final forecast that will be sent out to specific users and to the public”.
And now the question is: Where does this ‘expertise’ come from? If the forecast is not a model output, what is it then? What finally is an ‘expert forecast’?
From raw model outputs to ‘expert forecast’
About a month ago, Dan Satterfield published an interesting post (The Great Facebook Blizzard- Storms and Rumors of Storms), on his blog at the AGU Blogosphere where the question “Is Posting Raw Model Data A Mistake?” is raised. A comparative mention to a ‘model forecaster’ versus ‘a meteorologist’ indicate that forecasting is more than conveying model outputs.
Let’s take a hydrologic example, the one of the EDF developments to build a streamflow forecasting system (you can read more about their forecasting system here). Taking advantage of many years of practice in hydrological forecasting, developments to formalize forecast uncertainty began exploring human expertise and forecasters’ capacity to translate forecast uncertainty into statistical confidence intervals (Houdant, 2004).
The initial idea was to first of all train operational forecasters to give estimations of the 10th and 90th percentiles of streamflow forecasts (i.e., the values below which 10% and 90% of river flow observations fall, given that the system is reliable). Exercises were carried out to make forecasters express numerically their intrinsic perception of the uncertainty associated with a future river flow condition. They were asked questions like: “When you forecast 10 mm at a given place, what uncertainty do you associate with this value?”
In other words, forecasters were asked to no longer give a unique forecast value, but to provide scenarios, namely an ‘average’ scenario, a ‘low’ scenario (the 10th percentile), and a ‘high’ scenario (the 90th percentile). This way of expressing forecasts through quantiles or scenarios pushed forecasters to give a more formal indication of forecast uncertainty. The approach required ‘probability reasoning’. Forecasters had to provide confidence intervals for their forecasts. They had to be trained to give the 10th and 90th percentiles to answer to the question they were used to be asked by users, i.e., “what is the forecast peak flow of a basin or inflow to a reservoir?”
At EDF, the discussions about the implementation of a probabilistic system were conducted until 2005-2006, when it was then decided to make it the rule at the forecasting centers: from then on, they would always communicate forecast intervals/quantiles to the users, hoping that forecasters would be able to calibrate ‘subjectively’ the confidence intervals they were associating with their forecasts. The notion of ‘subjective probability’ in forecasts (Murphy and Dann, 1984), based on expertise applied by the forecaster, was introduced in the practice of operational forecasting (Garçon et al., 2009). Training and case-study analyses were considered as efficient means to help forecasters in ‘calibrating’ their subjective probabilities and avoid over-confidence (underestimation of total uncertainties). Illustrations of the role of human forecast expertise in producing and communicating uncertain forecasts can be seen here and here.
What interaction can we expect between model outputs and ‘expert forecasts’?
In an interview conducted with forecasters at EDF in Grenoble, their point of view was clear: to contribute in making human expert forecasts reliable and to facilitate the production of such forecasts in a routine way, it is essential to provide forecasters with objective probability forecasts (automatically produced by the probabilistic forecasting system). It was said: “to effectively enhance the ability of expertise, it is necessary to develop interactive tools that provide the forecaster the ability to simply change scenarios of precipitation and flow generated automatically: the forecaster must be able in a few ‘clicks’ to narrow the dispersion of the ensemble forecasts or to redirect it towards higher or lower values” (free translation & not ipsa verba).
We must then understand that an ‘expert forecast’ (in France, we say ‘prévision expertisée’) contains the forecaster’s best judgement about the upcoming situation. This may mean that at least one type of goodness (according to Murhpy’s definition in his 1993 paper), the Type I: Consistency (correspondence between forecasts and judgements) might be at a high level, although, as also reminded by Murphy, “since forecaster’s judgements are, by definition, internal to the forecaster and unavailable for explicitly evaluation, the degree of correspondence between judgements and forecasts cannot be assessed directly”.
One of the issues is actually to pin the process down and make it transparent: but is it nearly (im)possible?
We come back here to a point we once raised in a paper by asking ourselves and the reader if ‘communicating uncertainty in hydro-meteorological forecasts was mission impossible’. At that time, we concluded by saying that “from [our] experience, there is an optimistic temptation to bet on a negative answer: the mission is not impossible, at least not in its absolute terms, although the tasks to be executed might be difficult to accomplish”.
Faulkner et al. (2007) called for a translational discourse between science and professionals, so far this discourse has not even reached the stage of a toddler. The discussion is definitely not yet closed!
The recent post by Tom and the discussions that follow it show very clearly that ‘consistency’ can still be a full topic of investigation!
References: For stork population, see here; for forecast skill, see here.
April 25, 2014 at 15:43
The fundamental problem I have with forecasters making changes to probabilistic forecasts, whether they be hydrologic or meteorological, is that there is the presumption that the forecaster has more information available that the (objective) modeling system lacks. My question is what is the basis of the additional information that the forecaster possesses, lacking in the modeling system? If it is ‘objective’ in nature, it should be included in the forecast system. Otherwise, we get into a very dark world of ‘forecaster judgement’. My personal experience is that ‘forecaster judgement’ becomes nearly synonymous with ‘gut feeling’ and this is replete with pitfalls! Often the forecaster plays the game of odds, knowing that rarer events are less likely, so they hedge their bets, as it were. Namely, forecasters are more conservative in predicting extremes and the prediction of a more extreme event is reduced to something the forecaster is more comfortable with and which they feel is more likely to be ‘right’. This is especially true with single-valued deterministic forecasts. With a purely objectively based ensemble/probabilistic forecast, how do we include the added uncertainty introduced by forecaster judgement by altering the objectively generated probabilistic forecast? Does the forecaster chase his/her tail to get the forecast spread to their liking? This process is not reproducible and I believe we can see that the forecast process degenerates into a kind of “curve-fitting” exercise. In the end, my belief is that allowing a forecaster to alter an objectively based, say, ensemble hydrologic forecast is a fools errand. The skill of the forecaster should be spent quality controlling the inputs and proper model initializations and, afterward, with the analysis and interpretation of results and communication with end-users.
April 28, 2014 at 00:30
Early in my career I heard the quote (paraphrased) “Forecasting is like tending a campfire. Our natural instinct is to get in there and move logs around but there comes a point where the best thing is to leave it alone.”
This weekend I also saw a movie “Moneyball”, Brad Pitt and Philip Seymour Hoffman’s story of using statistics and modelling/data mining to draft together the best baseball team. Pitt’s character is bucking decades of tradition of “scouts” doing subjective analyses to determine the best mix of players. These analyses are based on experience and gut feel, whereas Pitt is proposing going strictly by a quantitative model created by a college student.
There is a scene where one of these scouts confronts Pitt:
Scout: “You don’t put a team together with a computer.”
Pitt: “No?”
Scout: “No. Baseball isn’t just numbers. It’s not science. If it was, anybody could do what we’re doing, but they can’t. They don’t know what we [human baseball scouts] know. They don’t have our experience and our intuition. You got a [data mining] kid in there that’s got a degree in economics from Yale. You got a scout here with 29 years of baseball experience. You’re listening to the wrong one. Now, there are intangibles that only baseball people understand. You’re discounting what scouts have done for 150 years? Even yourself?”
Pitt: “Adapt or die.”
April 28, 2014 at 14:06
I feel like navigating in dangerous waters between Thomas Adams’s Scylla and Tom Pagano’s Charybdis.
Thomas Adams questions the basis of the additional information that the forecaster possesses, lacking in the modelling system. If it is ‘objective’ in nature, it should be included in the forecast system. In the end, his belief is that allowing a forecaster to alter an objectively based, say, ensemble hydrological forecast is a “fools errand”.
Tom Pagano on the other hand argues that forecasting isn’t just numbers. It’s not science. If it was, anybody could do what we’re doing, but they can’t. They don’t know what we [human forecasters] know. They don’t have our experience and our intuition.
To Thomas A: There is n u m e r u o s additional information that is lacking in “the” forecast system: above all “objective” observations that for different reasons stay outside the model system(s). Some were made after the last computer run, but most are n e v e r included such as observations of precipitation, cloudiness, 2 m temperature and 10 m winds (except from ships out in the oceans). The current NWP systems just do not know about the current weather!
To Tom P: You may give credence to Thomas’s skepticism about the ”very dark world of ‘forecaster judgement’”. During my time both as forecaster at SMHI and scientist at ECMWF I found that about half of the forecasters’ intuitive “thumb rules” just didn’t work. But very few forecasters or scientist bother to find out and you rarely find papers on this matter. One exception is “Evaluating Forecasters’ Rules of Thumb: A Study of d(prog)/dt” by Thomas M. Hamill in “Weather and Forecasting” October 2003. See http://journals.ametsoc.org/doi/abs/10.1175/1520-0434(2003)018%3C0933%3AEFROTA%3E2.0.CO%3B2
To both: There is indeed “forecast experience” and ”forecast intuition” but we cannot bow to them just at face value. They must be tested to find out which are effective. Forecasters must also be educated (and re-educated) in what is called “intuitive statistics” which often has Bayesian leaning, see http://www.flame.org/~cdoswell/publications/humanwxfcst_04.pdf (and why not much else at http://www.flame.org/~cdoswell/)
Discharging all human forecasters will just shift all the difficult weather forecast contemplation and deliberations to the public.