Forecasting algal blooms in lakes and reservoirs
Contributed by: Trevor Page, Paul Smith, Keith Beven, Ian Jones, J Alex Elliott, Stephen C Maberly, Eleanor B Mackay, Mitzi De Ville and Heidrun Feuchtmayr
Why is it needed?
Forecasting algal blooms is an increasingly high priority for water managers to aid in reactive decision-making and to avoid dangerous and expensive bloom impacts. Algal blooms are a global problem affecting water resources, recreation and ecosystems. Toxic algal species such as cyanobacteria (more commonly known as blue-green algae) can kill pets and livestock and lead to human illness, while non-toxic species can affect water taste and odour and can block filters at water treatment works. In a world with a changing climate and continued nutrient enrichment, the proliferation of toxic algal species is likely to be more common such that forecasting when algal blooms are likely to occur has become more critical.
The prototype forecasting system
Predicting bloom occurrence can assist water managers in making decisions to mitigate their impact. These are of course “down-chain” forecasts; they require forecasting of the “downward” cascading effects of hydrometerological forecasts on water bodies which involves many additional complexities associated with the waterbodies and their catchments. Making forecasts of algal blooms in lakes is particularly difficult because the drivers of blooms are complex and are phenomena both external and internal to a lake. Critical external drivers, which ultimately control which algal species proliferate, are meteorological conditions such as solar radiation, air temperature and wind speed and the transfer of water and nutrients from catchments. We developed a prototype lake algal bloom forecasting system driven by forecasts from the European Centre for Medium range Weather Forecasts (ECMWF): see Page et al, 2018.
We tested a prototype 10-day ahead algal forecasting system built around a conceptual/physically-based lake phytoplankton model (Reynolds et. al., 1992) within an ensemble Kalman filter structure allowing the assimilation of in-lake measurements prior to the generation of forecasts. To generate forecasts the lake model was driven using an ensemble of downscaled meteorological forecasts based on medium range forecasts provided by ECMWF. The forecast skill of the system was assessed using pseudo-real time forecasting experiments based on historic data for two lakes (Windermere south basin and Esthwaite) in the English Lake District. These have different depths and nutrient regimes leading to differing responses to meteorological drivers and hence provide differing forecasting challenges. Daily observations of stratification and fortnightly observations of algal species and in-lake nutrient levels were assimilated with forecast skill assessed against these variables as well as water temperature and chlorophyll-a (Fig. 1).
How did it perform?
Forecast skill was variable for different periods of the year and different lakes. The most difficult periods to forecast were those when the physical and/or biological systems were most sensitive to small changes in driving inputs and/or parameters. For example, large forecast uncertainties resulted at times where lake stratification was weak and small input errors made a large difference to model estimates. Significant uncertainties were also propagated from relatively low frequency nutrient input data. Windermere required an ensemble size of between 100 and 200 members to achieve stable forecasts but Esthwaite required a larger ensemble size of around 400 members. Using these ensembles forecasts were an improvement on a “persistence forecast” out to about 4 or 5 days ahead and it was concluded that higher frequency measurements of chlorophyll-a and nutrient concentrations would help to improve forecasts if they become available in the future. It remains to be seen whether these additional observations would provide a significant improvement over a persistence forecast given that these new observations are likely to improve the persistence forecast itself.
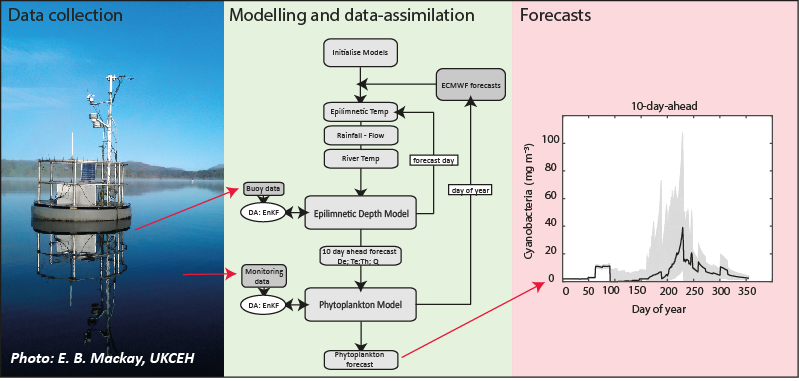
Figure 1 – Prototype algal bloom forecasting system: left pane shows a bouy recording high frequency data; centre pane is a schematic of the forecasting system and the right pane shows an example of concatenated forecasts with uncertainty as grey shaded area
Links
https://environmentagency.blog.gov.uk/2019/05/17/blue-green-algae-in-the-lake-district/
https://www.ceh.ac.uk/algal-blooms/bloomin-algae
https://www.walesonline.co.uk/news/wales-news/blue-green-algae-can-kill-14933565
https://freshwaterblog.net/2017/07/07/bloomin-algae-a-citizen-science-app-to-track-algal-blooms/
References
Page, T., Smith, P.J. Beven, K. J., Jones, I. D., Elliott, J. A., Maberly, S. C., Mackay, E. B., De Ville, M. & Feuchtmayr, H., 2018. Adaptive forecasting of phytoplankton communities, Water Research, (134), Pages 74-85: https://doi.org/10.1016/j.watres.2018.01.046.
Reynolds C.S., Irish A.E. and Elliott J.A. (2001). The ecological basis for simulating phytoplankton responses to environmental change (PROTECH). Ecol. Model., 140, 271–291.
0 comments