How good is my forecasting method? Some thoughts on forecast evaluation using cross-validation based on Australian experiences
Contributed by David Robertson, James Bennett and Andrew Schepen, members of the CSIRO Guest Columnist Team
As hydrological forecasting researchers, we are often excited when we develop new methods that lead to forecasts with smaller errors and/or more reliable uncertainty estimates. So how do we know whether a new method truly improves forecast performance?
The true test of any forecasting method is, of course, how it performs for real-time applications. But this is often not possible, and we have to rely on assessing retrospective forecasts (hindcasts). One problem with assessing retrospective forecasts is that performance metrics can be overly flattering if care is not taken to address the so called ‘artificial skill’ problem.
Artificial skill is most prominent when the same set of data is used to develop forecasting models and to assess forecast performance. Cross-validation is one way to reduce artificial skill. Cross-validation is essentially the separation of model development and forecast evaluation. Forecast models are developed using one set of data and forecast performance is evaluated on another (independent) set.
Ideally, model development and forecasting in cross-validation will closely resemble the approach to be used in real-time. For real-time forecasting, it’s usual to calibrate models using as much data as is available before a forecast is issued. In cross-validation, we want to assess our forecasts on as many forecasts as possible across a wide range of conditions. Leave-one-out methods, shown graphically in Figure 1, are able to maximise the data available for training our models. As we (often!) have short data records, ‘leave-one-out’ methods of cross-validation instantly appeal.
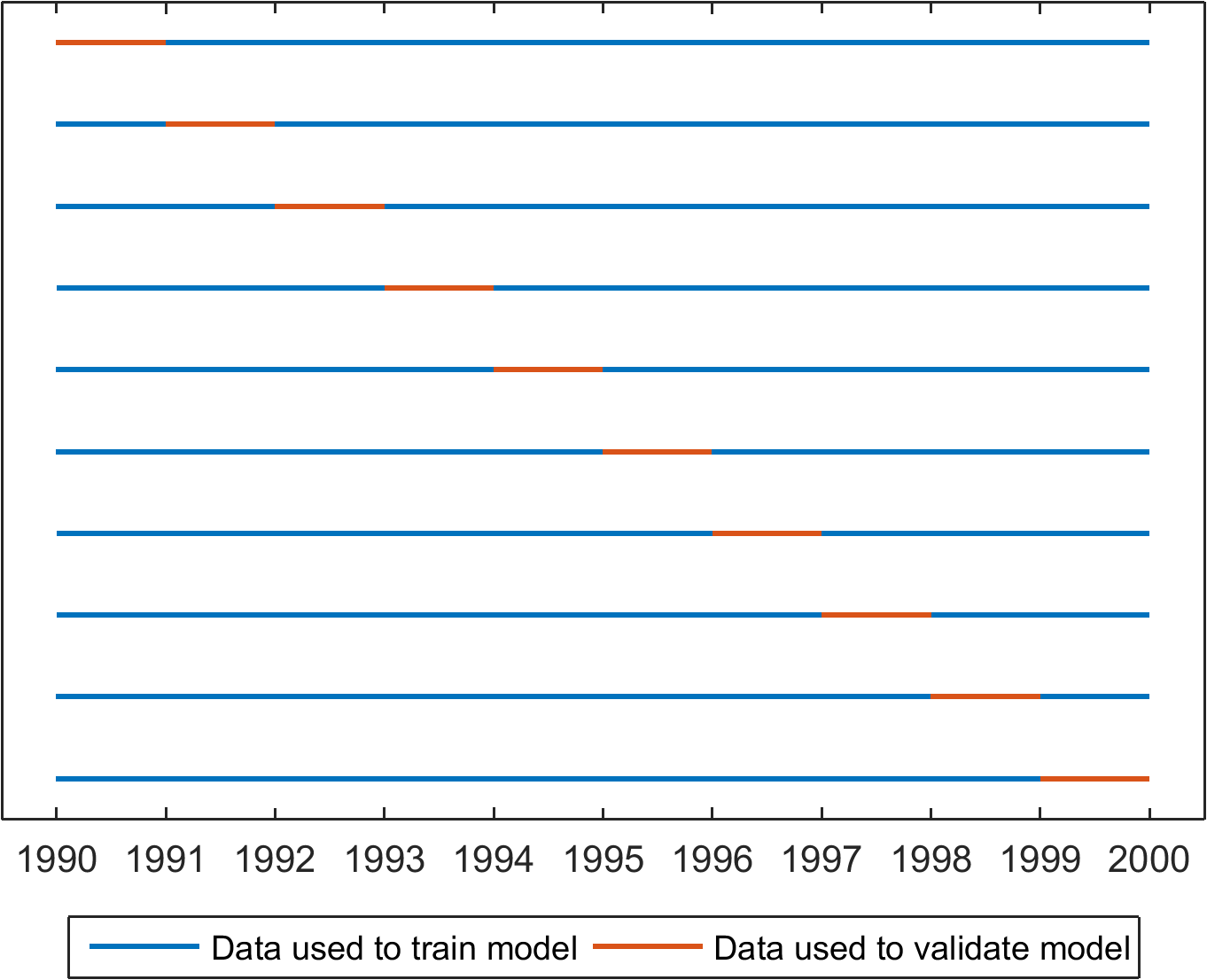
One problem with leave-one-out cross-validation schemes is that they allow us to build our model with data that are available after a forecast is issued. This is obviously not possible in real-time operations, and raises a thorny question: is it possible that data that occur after a forecast is issued can unfairly advantage our model?
For streamflow, the answer can be a resounding ‘yes’ (see Figure 2). Because catchments have memory, catchment conditions in say, 1995, can influence streamflows in 1996, 1997 or even longer. So if we are evaluating forecasts for 1995, we need to leave out not only data from 1995, but also 1996 (or more) when training our model.
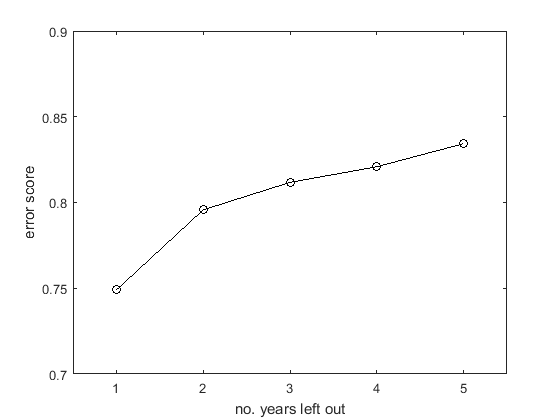
The next question then is: how much data do I need to leave out? Our experience in seasonal forecasting is that we need to omit at least two years afterwards. In most cases, a ‘leave-5-years-out’ cross-validation is adequate, and we use this routinely when evaluating seasonal streamflow forecasts (Figure 3).
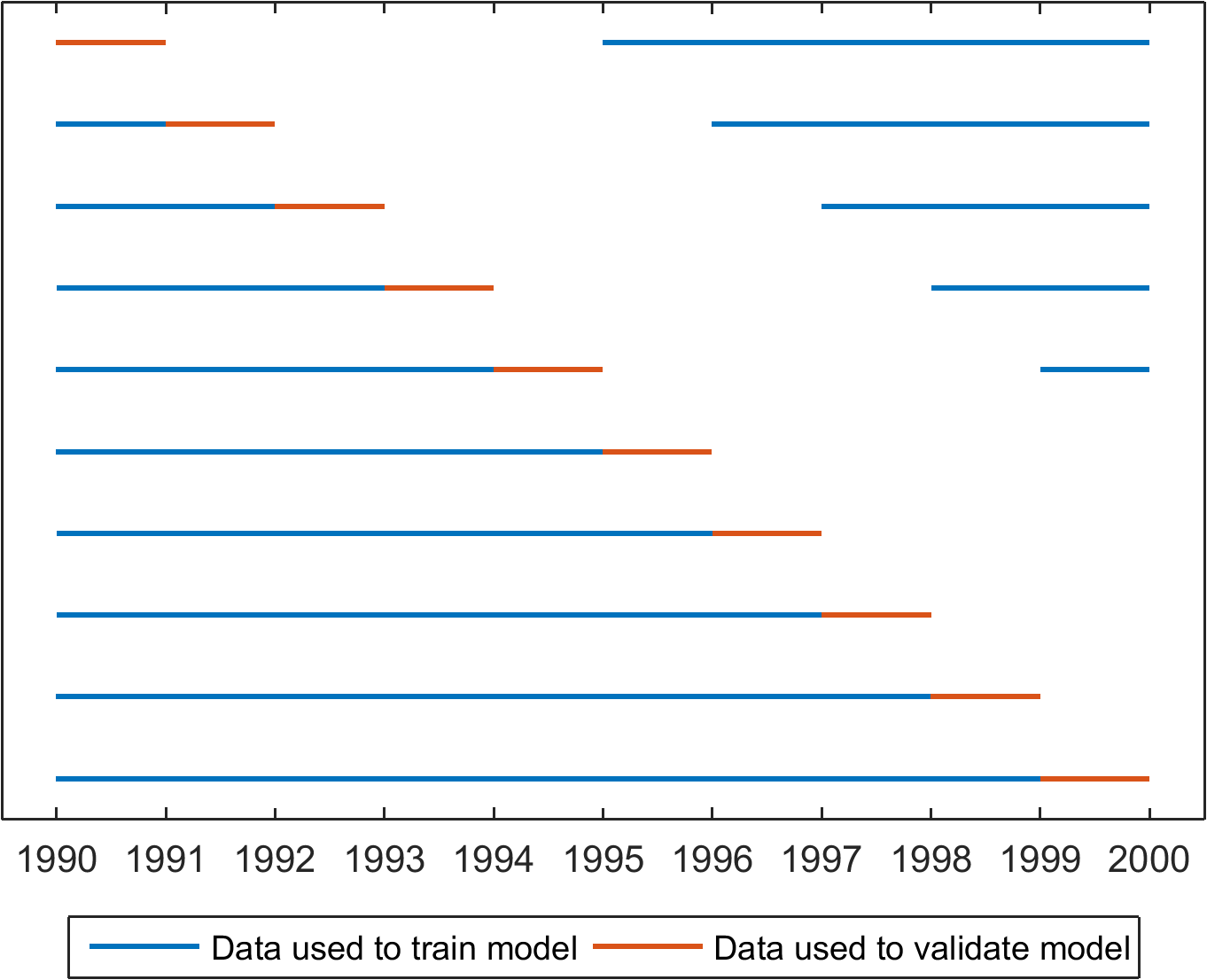
What else has tripped us up?
- A perennial issue is the need to cross-validate all elements of forecasting system development. Many forecasting techniques select predictors (e.g. climate indices) that are then used in another model (e.g. a regression) to predict streamflow. In such cases predictor selection and model parameters usually need to be cross-validated to avoid overstating forecast performance.
- Forgetting to cross-validate reference forecasts can unfairly disadvantage your forecast method. Remembering to cross-validate the reference forecast (e.g. climatology) is just as important as cross-validating forecasts.
Depending on how you look at it, proper cross-validation of forecasts can be a bruising or enlightening experience, especially if a model performs fantastically in initial testing and then falters under cross-validation. Cross-validation is an essential component of every hydrological forecaster’s toolbox and ensures our forecasts stand up to the test of real-time.
And now, over to you:
- What are your experiences with cross-validation?
- Do you use different cross-validation schemes to those described here?
- Do you have any suggestions/methods for cross-validation that make the most of available data without unfairly advantaging your forecasts?
March 8, 2016 at 11:03
Very nice post. Do you also consider the case of a centred window around the validation year (e.g. leave out 2 years before and after the validation year)?
Sometimes I also use a “random” cross-validation: split the data set randomly in two parts, use the first for model training/selection and the second for model testing (in the literature this is sometimes termed Monte Carlo cross-validiation or repeated-learning testing). This way, we can repeat the exercise as many times we want and also can have a look at the variability of e.g. the MSE estimate.
March 9, 2016 at 00:30
Thanks Simon. We don’t usually use a centred window. Centred windows mean that you omit data that precede a forecast by some time (e.g. a year) when building your model. In real-time you can generally use any data that are available before the forecast is issued to build/warm up your model (within certain limits – I realise there’s usually a slight lag while
data are ingested and prepared for use in any forecasting system). So we think it’s OK to use data preceding a forecast to build the model, hence the trailing window.
March 8, 2016 at 16:27
Excellent discussion, thanks! We have indeed found published work in which cross-validation steps were omitted, and on re-doing the work, the performance of the touted method fell apart. Also, as you note, sample size is a major issue with seasonal forecast post-processing, yet I feel it is not sufficiently discussed — another plus for your post.
And on that point: can you be sure that your loss of skill as you increase the years left out is not at least partly due to the shrinking training sample size?
March 9, 2016 at 00:34
Thanks Andy, and well spotted! No, we can’t be sure that’s not the effect of sampling size. (I should have also cross-validated climatology or something using the same leave-x-out schemes and plotted skill, but I didn’t have time before the post!) We know from other work in this region that some catchments there have very long memory – for example when we warm up a monthly water balance model it can sometimes take 10 years (!) for states to stabilize. These are extreme cases, but we hope they serve to illustrate the broader point.
March 8, 2016 at 19:27
Thanks for the very nice post! If the main consideration for the ‘leave-multiple-out’ approach is to avoid artificial skill created by system memory (e.g., for streamflow), does it mean it is still reasonable to use the standard ‘leave-one-out’ approach for other variables with less memory (e.g., precipitation), to avoid sample size issues as Andy mentioned above?
March 9, 2016 at 00:43
Hi Yuqiong – yes, you are quite right. Precipitation has much less memory than streamflow, and we often use shorter cross-validation windows when we validate precip forecasts. For example, when looking at NWP forecasts, we often have to deal with very short forecast archives (sometimes little more than 12 months), so we use leave-1-month-out when cross-validating our post-processing methods. However, if cross-validating variables with more persistence – e.g. sea surface temperature – we would generally use longer cross-validation windows.
March 11, 2016 at 13:27
Very nice post, with practical tips. Thanks! I have two questions in mind:
– if one focuses on skill scores, is it still (very) important to ensure no artificial skill with ‘N-years cross-validation’ since, in this case, not only the forecasts but also the reference will be considered with the same cross-validation procedure (and the score will finally reflect a relative gain instead of absolute values of performance)?
– how do you handle non-stationarity in time if you find any in your historic time series? When you say “In cross-validation, we want to assess our forecasts on as many forecasts as possible across a wide range of conditions”, can we consider “non-stationarity” a condition in many systems today that we have to handle when moving from model to real-time forecasting?
Thanks again for the post!
March 11, 2016 at 19:56
If we do not play with stochastic-based models and our choice is one of the great physically-based models – the case of non-stationarity is not a problem for us 🙂
March 15, 2016 at 00:25
Hi Helena – we do find that it is important for skill, as well as absolute performance scores, to undertake ‘N-years cross validation’. We find that our forecasting models are better able to learn from the fitting data than a reference such as climatology. This may be peculiar to Australian conditions where we experience strong multi-year climate cycles and the hydrological systems can persist climate signals for many years. Of course, the impact will depend on the adopted reference: using persistence as a reference will mean that the reference is unchanged under different cross-validation procedures.
Accounting for non-stationarity is a real challenge for retrospective forecast verification. When assessing the skill of forecasts, the effect of non-stationarity will be related to the choice of reference. An assumed stationary climatology reference will have poorer absolute performance under non-stationary conditions than more dynamic references such as persistence. We have found instances where forecast skill computed using a climatology reference is highly flattering primarily because the forecasting model can describe non-stationarity while the reference cannot.
March 11, 2016 at 19:51
Thank you for the nice post!
I think that core concept of different cross-validations techniques is generally about model parameters sustainability (“different conditions” are just a beautiful wrapper) investigation or (in popular machine learning terms) – avoiding model overfitting. And this approach is of great importance for modelers too (not only for forecasters 🙂 ) – great article from G. Thirel et al. (http://www.tandfonline.com/doi/abs/10.1080/02626667.2014.967248) summarized general concepts of hydrological models cross-validation in space-time dimension.
I think it will be suitable to expand validation periods to 2-3 years – it may lead to more robust parameters sets.
It is still open question – what the model parameters set (that you derived on different time periods) will provide the best runoff forecast. And maybe we have to use all available parameters sets for building our forecast in ensemble manner?
I really like your idea about “watershed memory” – it mostly corresponds with J. Evaristo et al. (http://www.nature.com/nature/journal/v525/n7567/abs/nature14983.html) research about water mechanics in watershed system based on isotope analysis. I will test this hypothesis in my future research.
March 16, 2016 at 16:16
As a “statistical” hydrologist I would like to ask a a rather practical (trivial?) question: How can we deal with temporal discontinuities (as induced by CV/LOO schemes)
when we work with deterministic models? Do we simply ignore training data blocks, which are too short? (e.g. the one year in LOO round 5 in Fig. 3 on the right side)
Thank you again for the very interesting column!
March 17, 2016 at 00:49
Hi Simon, excellent question. When working with hydrological models our cross-validation strategy is applied only to the streamflow data used in computing the objective function during model calibration. The model is run continuously for the entire period and therefore there are no temporal discontinuities. For robust forecast validation, long periods need to be left out because the model state variables will contain information from the validation period.